Italian National Agency for New Technologies, Energy and Sustainable Economic Development
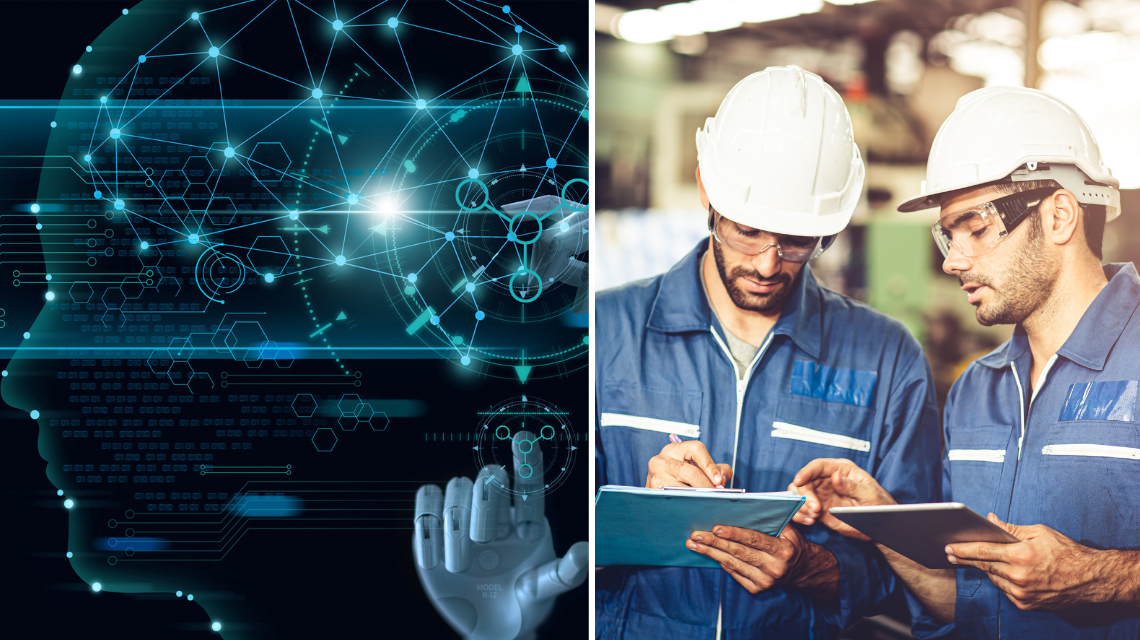
Innovation: ENEA, artificial intelligence to improve safety at workplaces
ENEA has developed AI tools to improve your workforce's safety and efficiency. The first tests proved effective in two different working contexts, a multinational pharmaceutical company and an Italian metalworking company. The research was published in the international J”ournal of Industrial Information Integration” and is part of the international project “Human-Centred Safety Crowd-Sensitive Indicators”, which includes ENEA (coordinator), Sapienza University of Rome, Middlesex University of London, the company Human Factors Everywhere and Inail, which provided the Italian funding quota[1].
“The methods and software tools we have developed are designed to ensure efficiency and safety in modern companies, where production processes are based on the interaction among people, physical instrumentation and technological components, including robots, drones, software and sensors. In technical jargon, we refer to cyber-socio-technical systems", explained Antonio De Nicola, researcher at the ENEA Laboratory for Analysis and Protection of Critical Infrastructures and co-author of the study with collegues Maria Luisa Villani, Francesco Costantino, Andrea Falegnami and Riccardo Patriarca of Sapienza University of Rome, Mark Sujan of Human Factors Everywhere and John Watt of Middlesex University.
The team of experts has defined a new resilience indicator[2] to capture the misalignment between formal procedures (Work-As-Imagined) and the work carried out by operators in factories and construction sites (Work-As-Done). “Efficiency and safety are often put at risk by this misalignment, as in reality there are multiple ways of 'seeing' the same work process and workers can change, out of necessity, what established by the protocol. But many of these changes can be potentially dangerous if you are in a power plant or on a construction site,” pointed out De Nicola.
To assess this indicator, a partially automated methodology was developed based on 'dynamic' questionnaires, to capture the differences between Work-As-Imagined and Work-As-Done, and on artificial intelligence, to analyse and quantify the gap between the two working methods.
In the company manufacturing semi-finished aluminum products, the indicator made it possible to identify the functions to be monitored more closely to ensure greater workplace safety. In the pharmaceutical production plant, also the main actions to be undertaken to improve the efficiency of operations were highlighted.
“Sometimes a misalignment between formal work procedures and actual work is 'regrettable' for failure to comply with safety procedures, while other times completing a work process actually requires deviation from protocol. In any case, these different perspectives can cause organizational tensions in the entire system and lead to a poor performance or even accidents due to failure to comply with safety procedures", said the ENEA researcher.
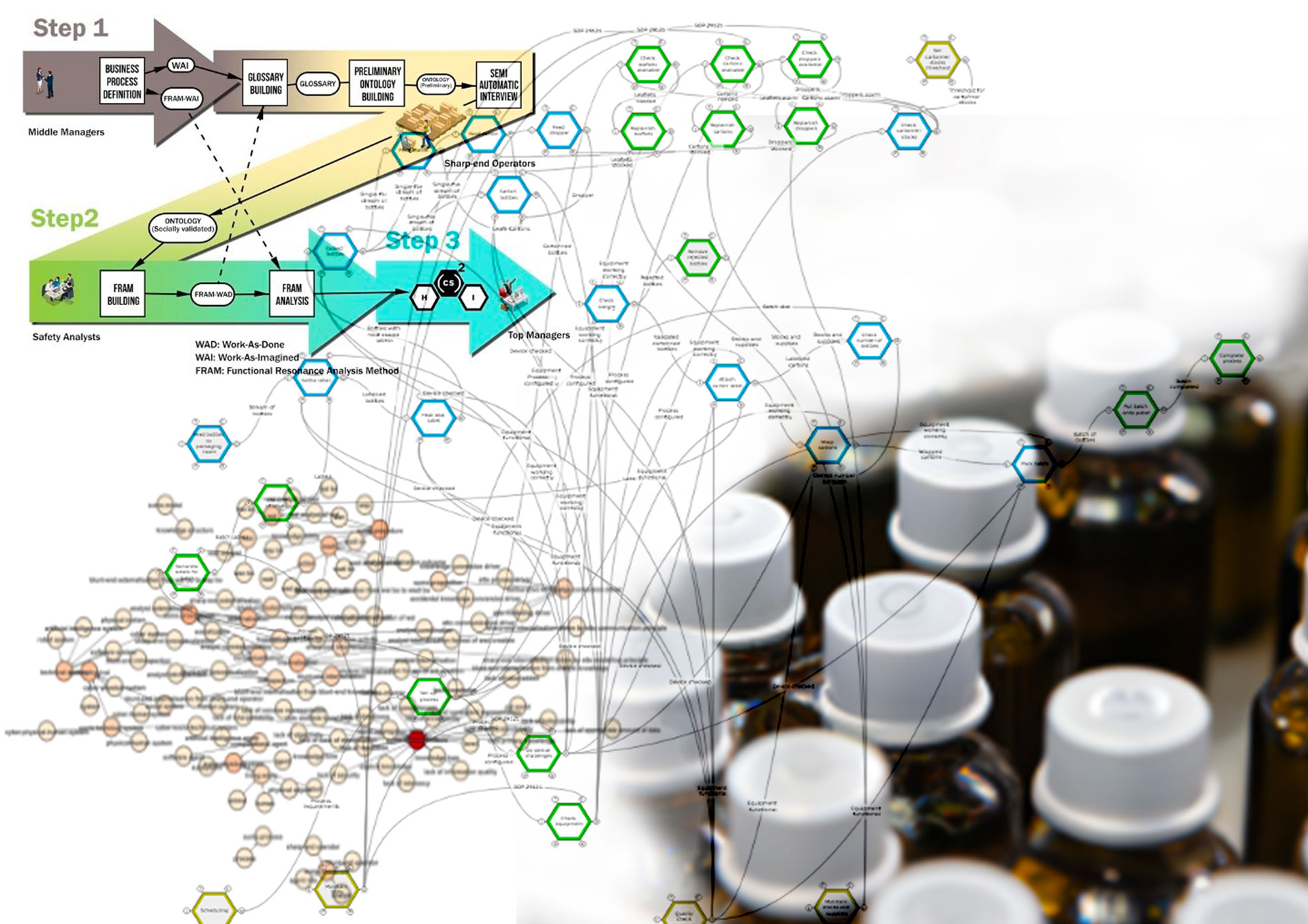